Imprecise ≠ Useless
Large Language Models are imprecise (for now). That doesn’t mean they’re useless.
LLMs are massive pattern recognition machines, trained on vast amounts of text data. When you enter a prompt, they analyze these patterns and predict what comes next, one word (or "token") at a time.
It's not about understanding in the human sense — it's about statistical probability. What word is most likely to follow? What phrase best continues this thought?
This approach means that LLMs are constantly trying to choose the next best word based on everything they’ve "seen" before. But it also means they can be unreliable. They might nail the perfect answer one moment, then spout nonsense the next.
In other words, they’re imprecise.
👉 But there are lots of tasks where precision *isn’t required.* 👈
Calculating the angles for a lunar landing? Very important.
Brainstorming alternate email subject lines? Not so important.
Every potential use case in your business exists somewhere on this spectrum, too.
Your external chatbot? More important.
Your internal knowledge base? Less important.
Good, Better, Best.
Let’s look at some examples.
Last time, we talked about using AI for analysis. For example, you could use ChatGPT to analyze your company performance data... so long as you were able to spot-check its calculations.
And depending on the importance of the data and that analysis, it’d be a pretty high risk-high reward use case. Accuracy would need to be pretty high for that analysis to be trustworthy and useful.
In contrast, what might imprecise analysis look like?
- Summarizing long articles or reports
- Detecting sentiment in customer reviews
- Categorizing and tagging content
- Identifying potential trends in data
- Compare and contrasting two documents
- Extracting key points from meeting transcripts
- Generating hypotheses for further research
- Identifying potential correlations or common themes in datasets
All of these tasks skew more qualitative than quantitative, more subjective than objective, more creative than conclusive.
In fact, we can apply this same thinking to all of the ways we use AI:
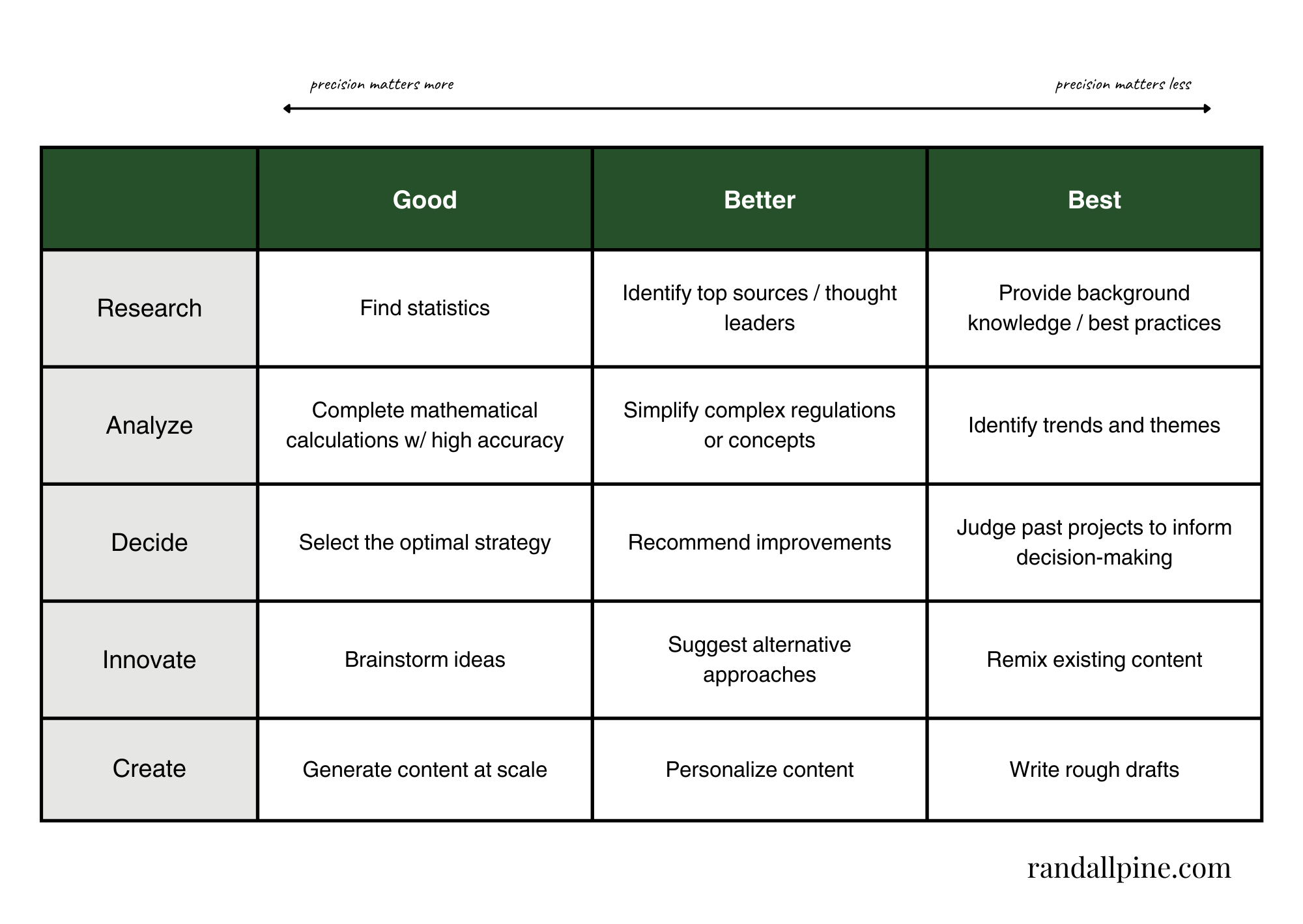
Perfection is so often the enemy of progress. Instead of dismissing LLMs when you encounter inaccuracy, consider a reframe:
What are your *most valuable* tasks where precision matters the *least*?
You're reading a preview! Subscribe for more growth, fewer growing pains.
Ever wonder how other growing companies make the tough decisions — like how much to spend on marketing, when it’s time to expand your org chart, or when and where to use AI?
Us, too. So we decided to create growthcurve: A newsletter dedicated to practical advice for growth without the growing pains.
Get frameworks, templates, and advice to smooth out the bumps along the way.
Sign up to receive growthcurve every other week.